AI/LLM
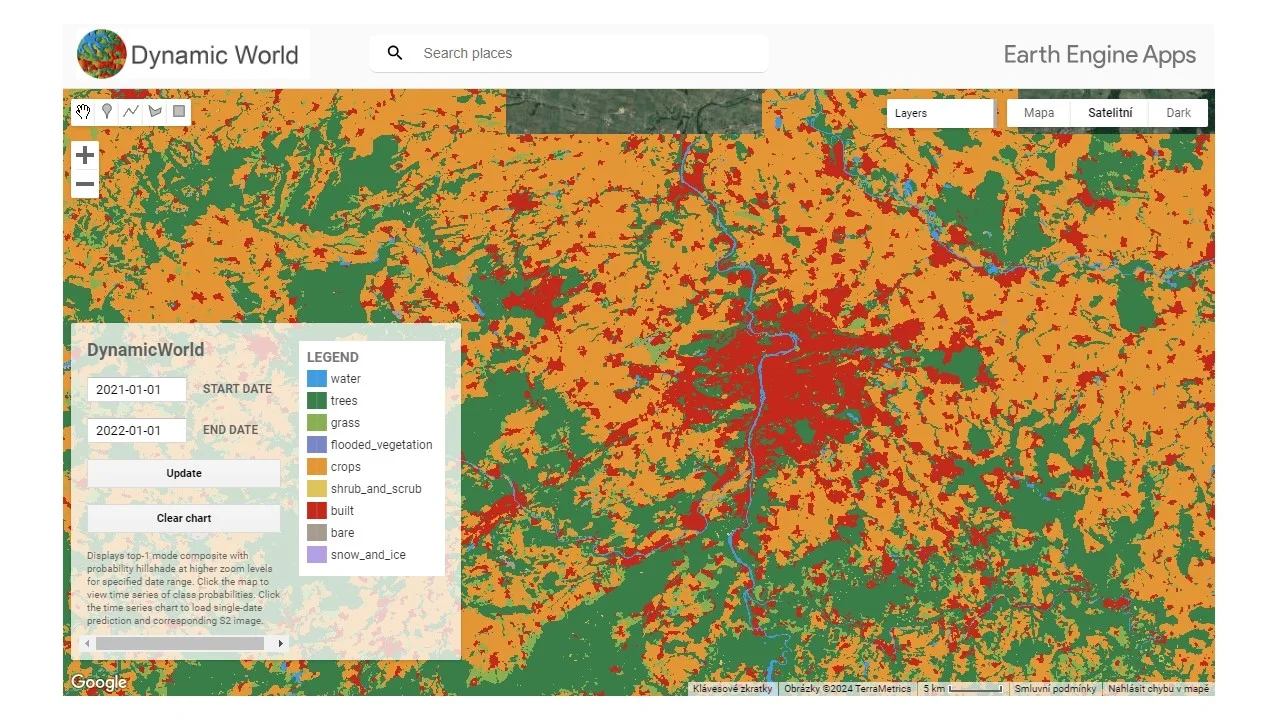
State-of-the-art
The application of modern artificial intelligence (AI) and large language models (LLMs) like ChatGPT in rural planning and land use management has gained considerable attention within the academic and professional communities. AI and machine learning (ML) techniques, particularly those utilizing satellite data from sources such as Sentinel-2 and Landsat, have become essential tools employed by large providers like Google Earth Engine. These tools facilitate detailed land-use analysis, allowing for precise detection of land-use changes over time. The ability to monitor and analyze these changes is critical for implementing timely and sustainable land management practices. Furthermore, AI-driven models are instrumental in exploring the interconnections between climate and land use. These models can predict the impacts of land-use changes on climate patterns and vice versa, thereby providing valuable information for developing strategies aimed at enhancing environmental sustainability and resilience.
LLMs offer substantial potential in the context of urban and rural planning by processing extensive textual datasets to generate insights and recommendations. Nevertheless, the deployment of current LLMs presents several challenges. A significant issue is the phenomenon of hallucination, where models generate incorrect or nonsensical information. Additionally, LLMs often lack access to critical local data, leading to outputs that are generic and not contextually specific. The high computational costs associated with LLMs further impede their widespread adoption. To mitigate these limitations, Retrieval-Augmented Generation (RAG) techniques have been increasingly utilized. RAG techniques enhance the extraction of relevant data from large textual datasets, thereby improving the accuracy and contextual relevance of the responses generated by LLMs.
PoliRuralPlus objectives for extension
We do foresee the need for work on the model level, too. But before we embark on that path, we will explore what may be done through working with existing models such as Gemini or GPT 4o by auto-engineering/enrichment of contexts.
Advancing the state of the art in AI and ML for rural planning necessitates a focus on developing localized land-use classification systems that do not rely exclusively on large providers such as Google. Localized AI models can provide more precise and context-specific land-use data, which is essential for effective community-level planning and management. Additionally, a comprehensive analysis of land-use development over time, in conjunction with its implications for climate change, can yield valuable insights into the dynamics of rural landscapes. Understanding these dynamics is crucial for enhancing the connections between rural and urban areas, such as examining the impact of rural land use on urban heat islands. These insights can inform more integrated and sustainable regional planning approaches, fostering a more cohesive interaction between rural and urban environments.
In the domain of LLMs, incorporating local factual data, such as geoJSON files, is a critical step towards improving the relevance and accuracy of the models' outputs. Ensuring the generation of truthful and original responses involves employing advanced techniques such as actor-critic models and iterative prompting procedures. These methods encourage the production of more nuanced and contextually appropriate responses. Additionally, integrating data from diverse sources, including text, tables, and RAGs, can further enrich the information processed by LLMs. Evaluating the economic feasibility of these approaches is essential to ensure that advanced AI and LLM technologies are accessible and practical for application in rural and urban planning contexts. This comprehensive approach aims to enhance the utility and accuracy of AI and LLM technologies, ultimately contributing to more effective and sustainable land-use management practices.
Modern AI and LLMs offer significant advancements in rural planning and land use management, but there are notable challenges and opportunities for improvement. Developing localized AI models and incorporating comprehensive land-use and climate change analyses are crucial steps in enhancing rural and urban planning. Similarly, refining LLMs to ensure the integration of local factual data and employing advanced techniques to generate accurate and context-specific responses are essential for improving their practical applicability. By addressing these challenges and leveraging the full potential of AI and LLM technologies, it is possible to achieve more effective and sustainable land-use management and planning practices.
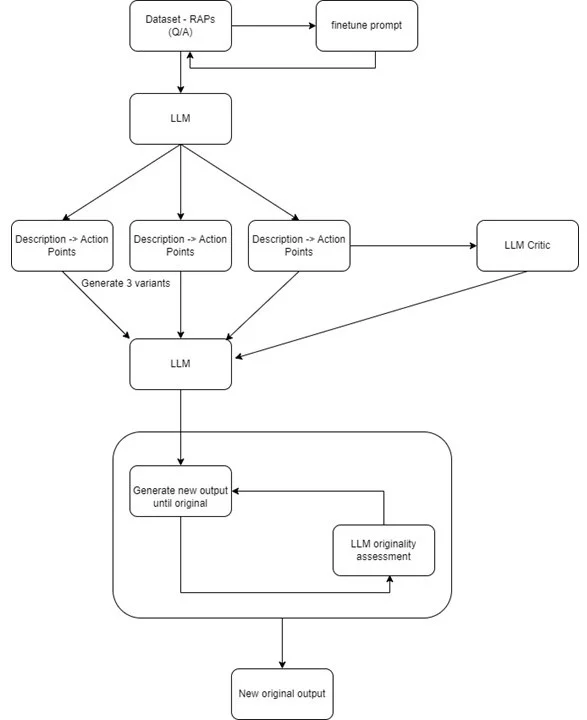